Adaptive Computing
NREL's adaptive computing research aims to reduce the computational and financial resources needed to address problems in engineering optimization and model training for multi-fidelity simulations.
Adaptive computing enables:
- Catalytic upgrading — surrogate modeling through integration with virtual engineering
- Electric grid reliability — machine-learning-based electrical controllers that learn on the fly from simulations of the electrical demands of communities of buildings.
Our adaptive computing research addresses engineering design optimization and model training for multi-fidelity simulations subject to resource constraints (use of computational and financial resources).
Simulations play a key role in engineering design, but often high-fidelity simulations that provide an accurate representation of physics require too much computational resources for routine use. Low-fidelity models—less expensive to perform—are less accurate.
Multi-Fidelity Modeling Framework
We propose a framework for performing multi-fidelity modeling, which learns trends from many low-fidelity simulations and uses a limited number of high-fidelity simulations to correct the low-fidelity simulations. This technique is being applied to discover novel materials for solar panels, develop efficient HVAC controls for buildings, and create next-generation biofuels.
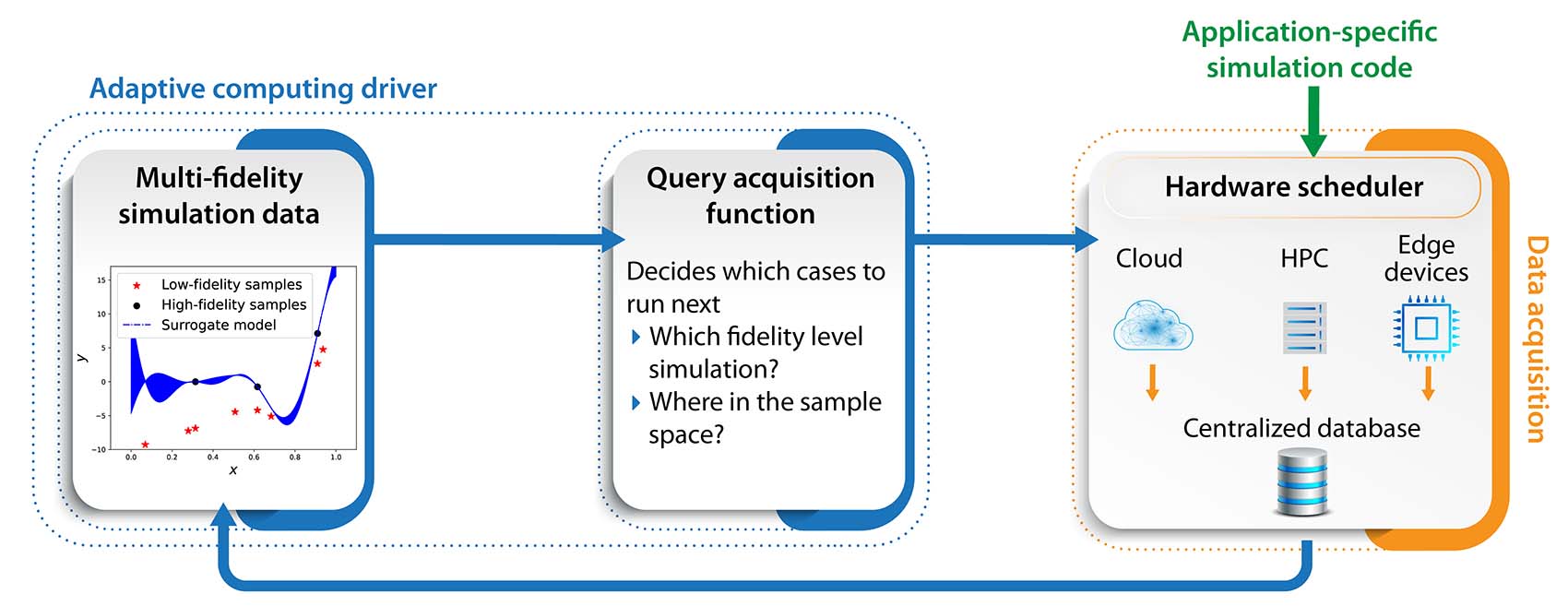
Data and Tools
G2Aero Software: Aerodynamic Shape Parametrization Using Separable Shape Sensors
Biomass Feedstock Conversion Interface Handling Computational Models
High-Fidelity Simulation Codes for Industrial Decarbonization
Combustion Simulations for Sustainable Aviation Fuels End Use
Virtual Engineering of Biofuels Software
PVade: Photovoltaic Aerodynamic Design Engineering Software
Development Team
NREL's adaptive computing development team includes Marc Day, Olga Doronina, Hilary Egan, Kevin Griffin, Marc Henry de Frahan, Ryan King, Juli Mueller, Jibo Sanyal, Deepthi Vaidhynathan, and Dylan Wald.
Contacts
Share
Last Updated April 10, 2025